Invited Speakers

Dr. Wei Chen
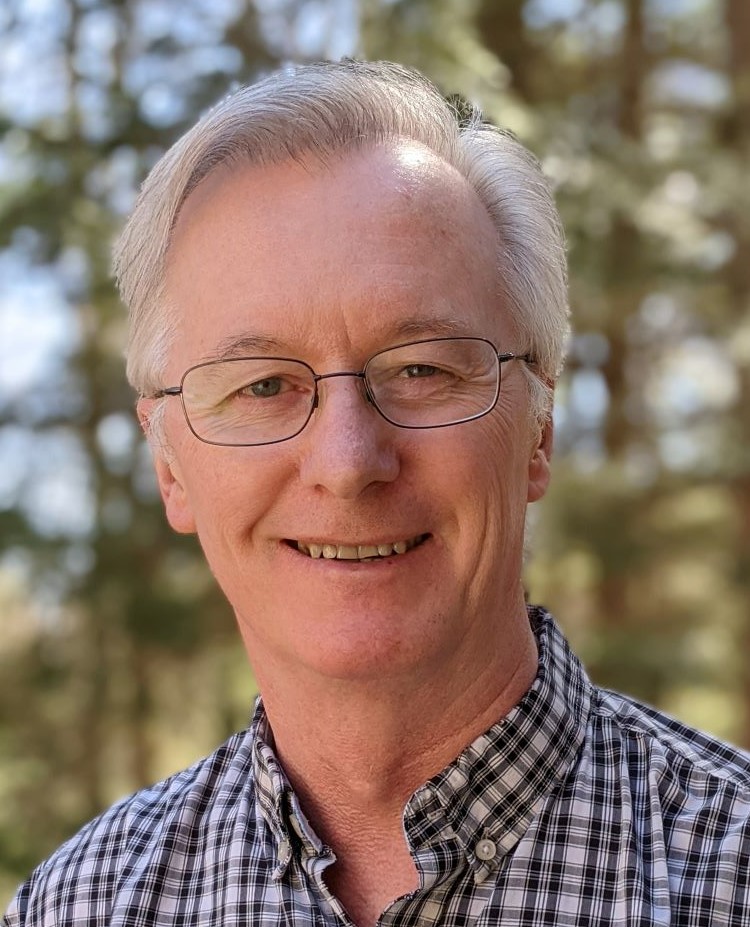
Dr. Chris Hill
Ansys Inc.
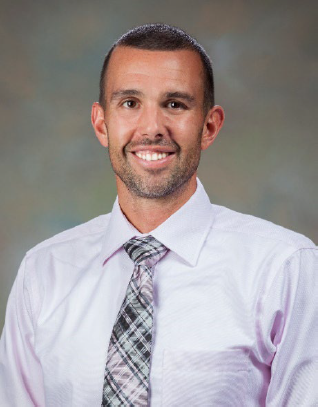
Dr. Justin Newcomer
Sandia National Laboratories

Dr. John G. Michopoulos
US Naval Research Laboratory
Plenary Presentation Abstracts
Presenter's Bio: Dr. Wei Chen is the Wilson-Cook Professor in Engineering Design and Chair of Department of Mechanical Engineering at Northwestern University. Directing the Integrated DEsign Automation Laboratory (IDEAL- http://ideal.mech.northwestern.edu/), her current research involves the use of statistical inference, machine learning, and uncertainty quantification techniques for design of emerging materials systems including microstructural materials, metamaterials and programmable materials. She serves as the Design Thrust lead for the newly funded NSF Engineering Research Center (ERC) on Hybrid Autonomous Manufacturing, Moving from Evolution to Revolution (HAMMER), where she works on digital twin systems for concurrent materials and manufacturing process design. Dr. Chen is an elected member of the National Academy of Engineering (NAE) and currently serves as the President of the International Society of Structural and Multidisciplinary Design (ISSMO). She served as Editor-in-chief of the ASME Journal of Mechanical Design and the Chair of the ASME Design Engineering Division (DED). Dr. Chen is the recipient of the 2022 Engineering Science Medal from the Society of Engineering Science (SES), ASME Pi Tau Sigma Charles Russ Richards Memorial Award (2021), ASME Design Automation Award (2015), Intelligent Optimal Design Prize (2005), ASME Pi Tau Sigma Gold Medal achievement award (1998), and the NSF Faculty Career Award (1996). She received her Ph.D. from the Georgia Institute of Technology in 1995.
Abstract: Concurrent materials, geometry and manufacturing process optimization involves many computational challenges such as high-dimensionality associated with location dependency, material heterogeneity, multi-modal information, and nonlinear material behaviors such as large deformations and plasticity. The recent growth of using physics-based machine learning creates opportunities for incorporating data-driven methodologies with physical models into design. Furthermore, digital twin is an emerging technology in the era of Industry 4.0 that holds promises for real time optimization of manufacturing processes and quality control. We will present in this talk a digital twin framework that integrates materials modeling, part-scale manufacturing simulation and mechanics analysis, as well as state-of-the-art data science techniques to achieve off-line surrogate modeling and model uncertainty quantification and on-line predictive model control. To support materials-aware product and process design, techniques of microstructure characterization and reconstruction, machine learning of materials laws, differentiable simulation based optimization, and deep-learning based computer vision metrology will be introduced.
Presenter's Bio: Dr. Chris Hill has a B.S.c in Natural Philosophy and Mathematics from the University of Glasgow, Scotland. He holds a Ph.D. from the University of Cambridge Engineering Department, Cambridge, England, in acoustics and fluid mechanics. His research interests include the interaction between hydrodynamics and acoustics, boundary layer transition and receptivity, flow control, adjoint methods, optimization, lattice Boltzmann methods, finite volume methods, and machine learning for CFD. Dr. Hill is the original author of the Ansys Fluent adjoint solver. He has spent time at NASA Ames Research Center as a Post Doc. and Stanford Center for Turbulence Research as a Senior Fellow. For the last 25 years he has worked at Fluent and Ansys where he is currently a Technical Fellow and Chief Technologist for Fluids.
Abstract:
Presenter's Bio: As a Principal Scientist of Materials Innovation in the Materials Science and Technology Division and as the head of Computational Multiphysics Systems Lab (CMSL), of the Center for Material Physics and Technology at the US Naval Research Laboratory (US-NRL), Dr. Michopoulos executes and oversees multi-physics modeling and simulation efforts and computational sciences research and development, operations and initiatives at CMSL. Some of his major initiatives include research and development on linking material performance to material processing via data and specification driven methodologies, additive and hybrid manufacturing process and material modeling, mechatronic/robotic data-driven characterization of continua, multiphysics design optimization and meta-computing. He is a member of the editorial board of several scientific journals and is member of the program committee of several international conferences and has chaired several of them. He has served in the executive committee of the Computers and Information in engineering division of the ASME. His technical work and leadership have been recognized by several national and international honors, including the 2015 Excellence in Research award and the 2022 Lifetime Achievement award by ASME’s CIE division, the 2015 Innovator Award by Wolfram Inc., and the 2013 “P.S. Theocaris” award for excellence by the National Academy of Athens. He has authored and co-authored more than 370 publications and patents. Dr. Michopoulos holds a Diploma/M.Sc. in Civil Engineering and a Ph.D. in Applied Mathematics and Mechanics from the National Technical University of Athens and has pursued post-doctoral studies at Lehigh University on computational multi-field modeling of continua and Fracture Mechanics.
Abstract: An overview of the development, impact and future potential of data-driven digital twins (D3T) will be presented from the perspective and context of material science. First the delineation between a model and D3T will be introduced in the context of epistemology and systems theory. Then, an existential and ontological framework of D3Ts will be presented in terms of the fundamental questions and needs that make their utility practical. Several historical examples of D3Ts along with custom-developed multi-degree of freedom robotic testing systems for generating data that were developed since the 1960s at the U.S.-Naval Research Laboratory involving composite materials will be presented. Finally, an overview of the drivers that push the extension of D3Ts beyond their present state, along with potential examples of future D3Ts in the context of material and structural systems will close the presentation.
Presenter's Bio: Dr. Justin Newcomer is the Senior Manager and Deputy Program Executive for Sandia National Laboratories’ Advanced Simulation and Computing (ASC) Program. In this role, Justin assures the execution of Sandia’s ASC program which provides state-of-the-art computer simulation capabilities as part of NNSA’s mission to extend the lifetime of nuclear weapons in the stockpile without underground testing. In this role, Justin also leads an initiative to accelerate the use of artificial intelligence (AI) and machine learning (ML) for Sandia’s Nuclear Deterrence mission.
Previously, Justin was the Senior Manager of Sandia’s Statistics and Human Systems Group which provides deep and differentiating expertise in statistics, ML, applied cognitive science, and human factors broadly across the laboratories. From 2019 to 2020, Justin led the Machine Intelligence Department where he initiated and led several AI and ML research activities for Sandia. From 2016 to 2019, Justin led Sandia’s Statistical Sciences Department and was mathematical statistician in that department from 2009 to 2016. His technical work has supported many national security and defense programs by providing statistical expertise on test planning, design of experiments, and statistical methodology development for engineering applications.
Justin completed Sandia’s Nuclear Weapons Internship Program in 2013 and was Sandia’s Laboratories Director Nuclear Weapon Red Team Chairman from 2013 to 2015. Justin received a Ph.D. and master’s degree in statistics from the University of Maryland Baltimore County and bachelor’s degrees in mathematics and computer science from West Chester University.
Abstract:
This talk will explore the spectrum of research, development, and application of AI technologies at Sandia and the opportunities and challenges in realizing AI’s full potential. The talk will start with an exploration several of the AI research and development efforts at Sandia to address unique national security challenges. The talk will then transition to a discussion on the challenges with adoption of these technologies and the roadblocks we face in realizing the full potential of AI for Sandia’s key national security missions. Finally, the talk will conclude with a number of examples of early successes and our strategy for further accelerating the use of these technologies at Sandia.